AI will impact every area of our lives, but it will have a disproportionate impact on healthcare and biology, moving healthcare from reactive and generalized, to proactive and personalized. We are excited to share our updated thesis on AI’s transformative impact on the healthcare industry, released to coincide with global heathcare gathering HLTH 2022.
In the early days of the pandemic, we anticipated advances in healthcare unfolding in two waves of innovation. Wave 1 is defined by broad digital adoption, creating the data, digital infrastructure, and behavioural foundations for the deep tech innovations of Wave 2, which would be primarily driven by AI.
Two years later it is clear that Wave 2 has begun to arrive. Previously siloed healthcare data is becoming more accessible, and AI is well-positioned to responsibly leverage multi-modal healthcare data and combine it real-time with secure flows of clinically relevant data to improve health outcomes. AI powered healthcare is entering the mainstream.
In this report, we share our perspective on the areas where we expect to see AI generate outsized value including innovations in health data sets, genomics and precision medicine, generative AI applied to biology, and AI-enabled sleep solutions.
Radical has invested in 10 healthcare AI companies. These teams are successfully deploying healthcare innovations in the clinical setting, bringing new biology assets to the market and creating significant efficiencies for their customers. In this report, several of these founders share their insights about deploying healthcare AI innovations in the real world and commercializing their technology.
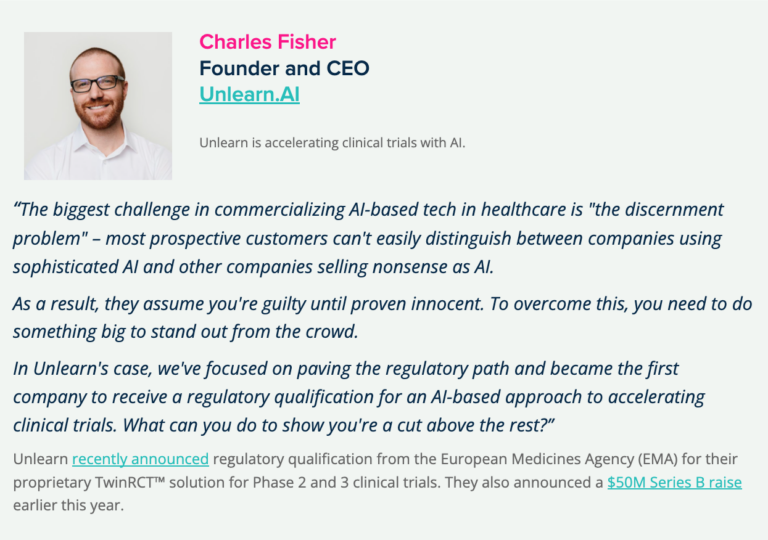
We believe the healthcare AI revolution has just begun. Please reach out to us if you are building in this space.
Stay tuned for more insights and continued work on our De-mystifying Healthcare AI series.
AI News This Week
-
Next Big Things in Tech 2022 (Fast Company)
A panel of 22 Fast Company editors and writers selected this year’s Next Big Things in Tech winners. The award identifies technology companies that are pioneering new approaches predicted to transform industry over the next few years. Radical portfolio company Cohere was recognized in the AI and Data category “for bringing natural language processing to the little guy.”
-
Waabi unveils its first-generation self-driving truck (TechCrunch)
Radical portfolio company Waabi has unveiled its first generation of trucks that are purpose-built for OEM integration. The trucks are powered by the Waabi Driver, a combination of Waabi’s software and hardware (sensors and compute). The Waabi Driver is designed to be integrated into vehicles at the factory level with no interruption to an OEM’s assembly line.
-
The 13 most promising early-stage artificial-intelligence startups of 2022, according to VCs (Business Insider – Subscription may be required)
There is a future in healthcare with faster clinical trials, smaller control groups, and an ability to deliver findings for each patient. Radical Ventures portfolio company Unlearn is doing this by developing digital twins – AI programs to simulate how a patient would behave if they were part of a control group. The company has teamed up with several drugmakers to realize this future of faster and cheaper clinical trials using AI-generated control test cohorts. Big pharmaceutical companies are spending roughly double what they did about 20 years ago on drug development and Unlearn can transform the costly drug-development process.
-
Stanford debuts first AI benchmark to help understand LLMs (VentureBeat)
Also, on Radical portfolio company Cohere, a new study from Stanford’s The Center for Research on Foundation Models (CRFM), has shown that the Cohere xlarge language model achieves higher accuracy than a number of well-known models which are 3x larger, including GPT-3, Jurassic-1 Jumbo, and BLOOM. Cohere’s model is the second highest performing of those commercially available, and the highest performing of any independent company.
-
How online mobs act like flocks of birds (Noema)
A growing body of research suggests that human behaviour on social media — coordinated activism, information cascades, harassment mobs — bears striking similarity to “murmurations” or “emergent behaviour” in nature. A flock of birds or a school of fish, for example, act as cohesive units without hierarchical leadership. Online moderators currently pay attention to content substance to weed out harmful instances. This framework may miss opportunities to examine sentiment structures. AI may help define structural analyses and inform design change. In the past, AI has been applied to animal murmurations to better understand behaviours such as swirling. Drawing on computational biology and complex systems we may be able to re-envision our social media experience in a more productive, content-agnostic way.
Radical Reads is edited by Leah Morris (Senior Director, Velocity Program, Radical Ventures).