The pace of innovation in AI today is blistering. In the last few weeks, between open-source momentum (hello, AI Woodstock!), autonomous agent hype, and the Google’s AI research restructuring — just to name a few — there’s been a lot going on.
In what feels like a constant AI news cycle, let’s take a step back and look at the big picture Here are 10 charts that start to make sense of AI today.
#1: A battle is underway between closed and open models.
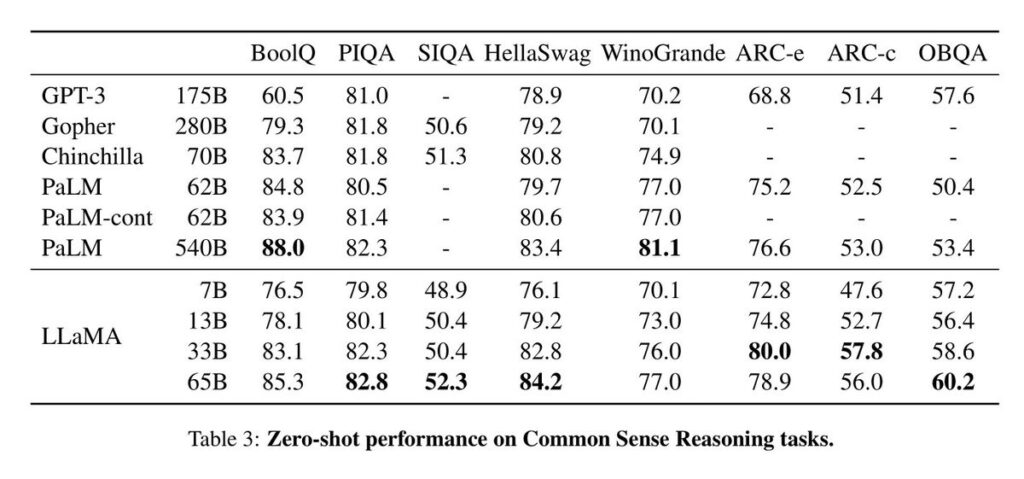
Source: Meta AI, “LLaMA: Open and Efficient Foundation Language Models”
This may be the most important trend in AI right now. Some are calling this AI’s Linux, or Android, moment.
Open-source models have become a critical part of the AI ecosystem, particularly after the introduction of Stable Diffusion last summer. Previously, however, open LLMs lagged in performance. As a result, the prevailing wisdom has been that the highest-performing language models were behind APIs.
No longer. New open LLMs are roiling the ecosystem. Meta AI kicked off the cycle with LLaMA, a family of models licensed for research use that rivaled GPT-3 and others in terms of performance (results above). Then, Stanford released Alpaca, a fine-tuned version of LLaMA, which reportedly can be reproduced for only $600. After that came the stampede of the camelids: Databricks’ Dolly and Dolly 2.0, the latter of which is importantly licensed for commercial use; Stability’s StableLM; GPT4ALL; and Together’s RedPajama.
The implications of the shift towards open LLMs are significant. As Stable Diffusion illustrated in the image domain, open models enable mass experimentation and innovation at the application layer; we may now see the same in LLMs as the cost of experimentation drops. Open models may also provide a complement, if not a challenge, to the businesses of closed, API-based models. Finally, open models pose new governance challenges, as outputs become harder to manage and misuse harder to track.
#2: After years of “bigger is better,” smaller, distilled models may be the next (little) big thing.
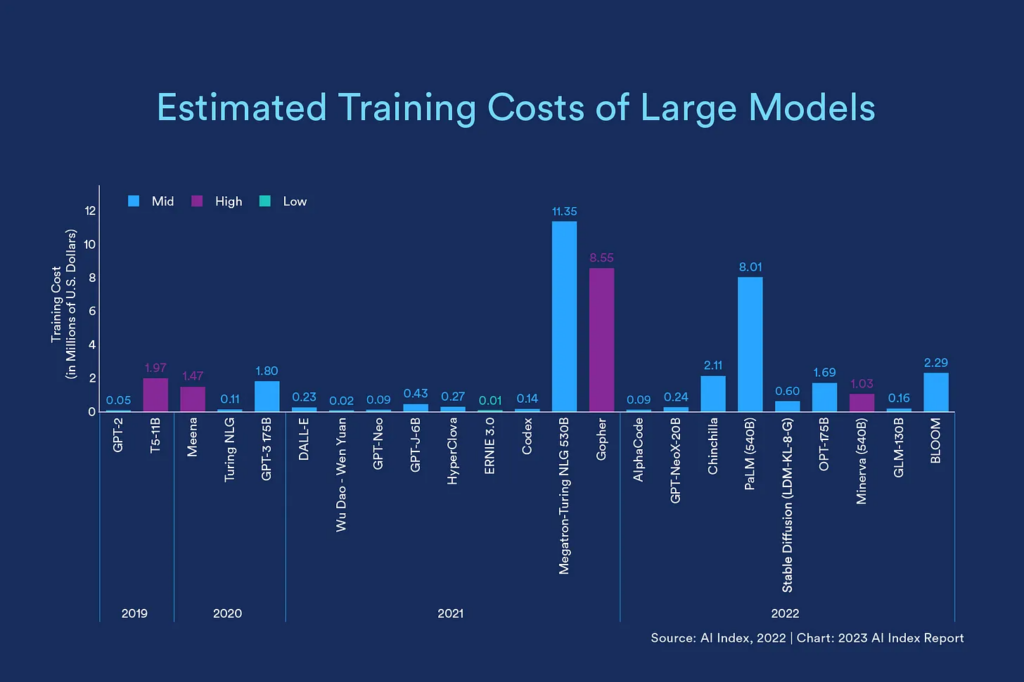
Source: Stanford HAI AI Index 2023
Richard Sutton’s “bitter lesson” described one dominant point of view in the ML research community over the past decade: the recipe for progress in AI was bigger models, trained on more data, benefiting from the tailwinds of Moore’s Law. And indeed, ever-larger language models have yielded impressive results.
However, these large models are very expensive to build and deploy. The chart above documents the increasing cost since 2019 of training large models. Inference costs, of course, are also material for models of this scale. As a result, there is growing momentum around “distilled” smaller models, potentially fine-tuned on specific domains.
There are other noneconomic advantages to distilled models, like latency. Small models fine-tuned for specific enterprise use cases are not only cheaper and faster to train and run, but they also enable companies to avoiding sending sensitive information through an API by running on-premise or even on-device. Expect more innovation in this domain.
#3: Data is the new oil, and the global AI economy still runs on it.
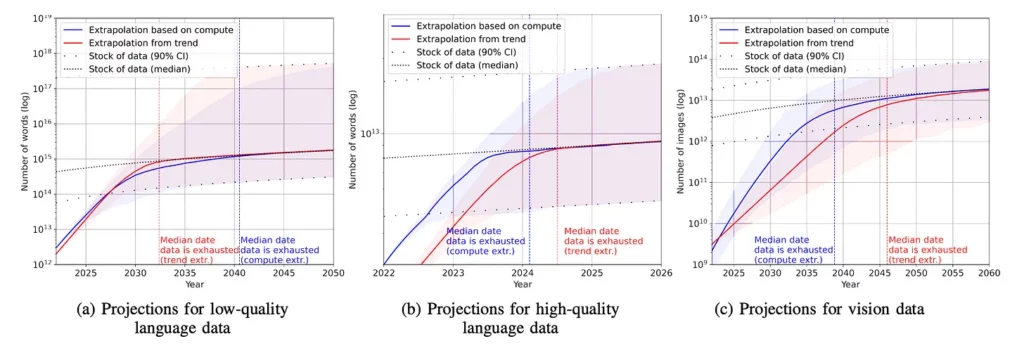
Source: “Will we run out of data? An analysis of the limits of scaling datasets in Machine Learning,” Arxiv
DeepMind’s landmark Chinchilla research underscored the importance of scaling training datasets proportionally to model size for performance. However, several analyses have indicated that we may be running out of training data, both in language and vision. The chart above provides projections for language data (both high and low quality), estimating that the stock of high-quality language data will be exhausted by 2026.
Bumping up against data limitations has major implications for NLP research. It could also provide a catalyst for data efficiency innovation and tailwinds for the synthetic data market.
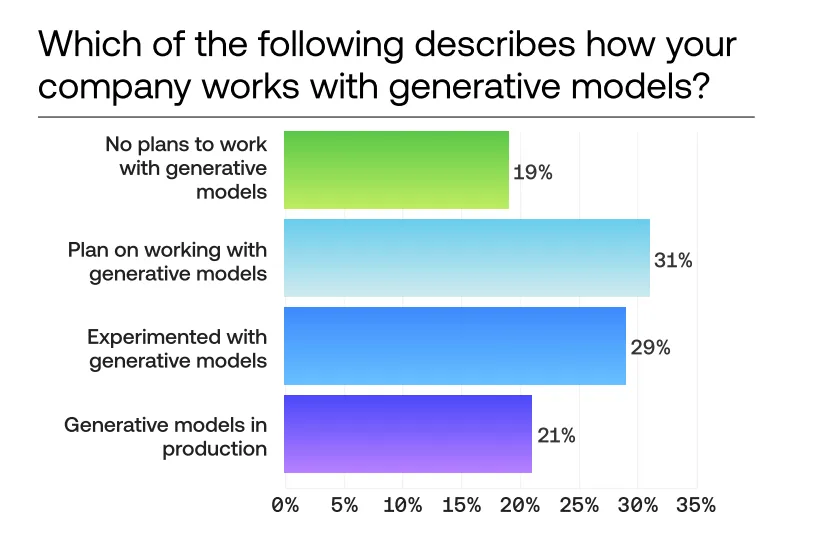
Source: Scale AI 2023 AI Readiness Report
After more than 10 years of commercialization, enterprises are still figuring out how to use AI.
In 2022, McKinsey found that only 50% of enterprises had adopted AI in at least one business unit, and that usage had actually gone down since 2019. Moreover, despite generative AI’s big boom in 2023, most enterprises are still just musing or experimenting: only 20% of enterprises recently surveyed by Scale AI indicate they are running generative models in production.
Under-penetration in the enterprise is both an opportunity and challenge. The opportunity, of course, is that there’s meaningful headroom for enterprise AI adoption, particularly when it comes to generative models. At the same time, enterprise AI applications need to deliver real business value to drive consistent usage once the headlines pass. This is especially true in 2023 macro, as still-high interest rates and sluggish growth force cost reductions across the Fortune 500, including in enterprise software budgets.
#5: At the same time, we’ve seen the breakout consumer moment for AI. What happens now?
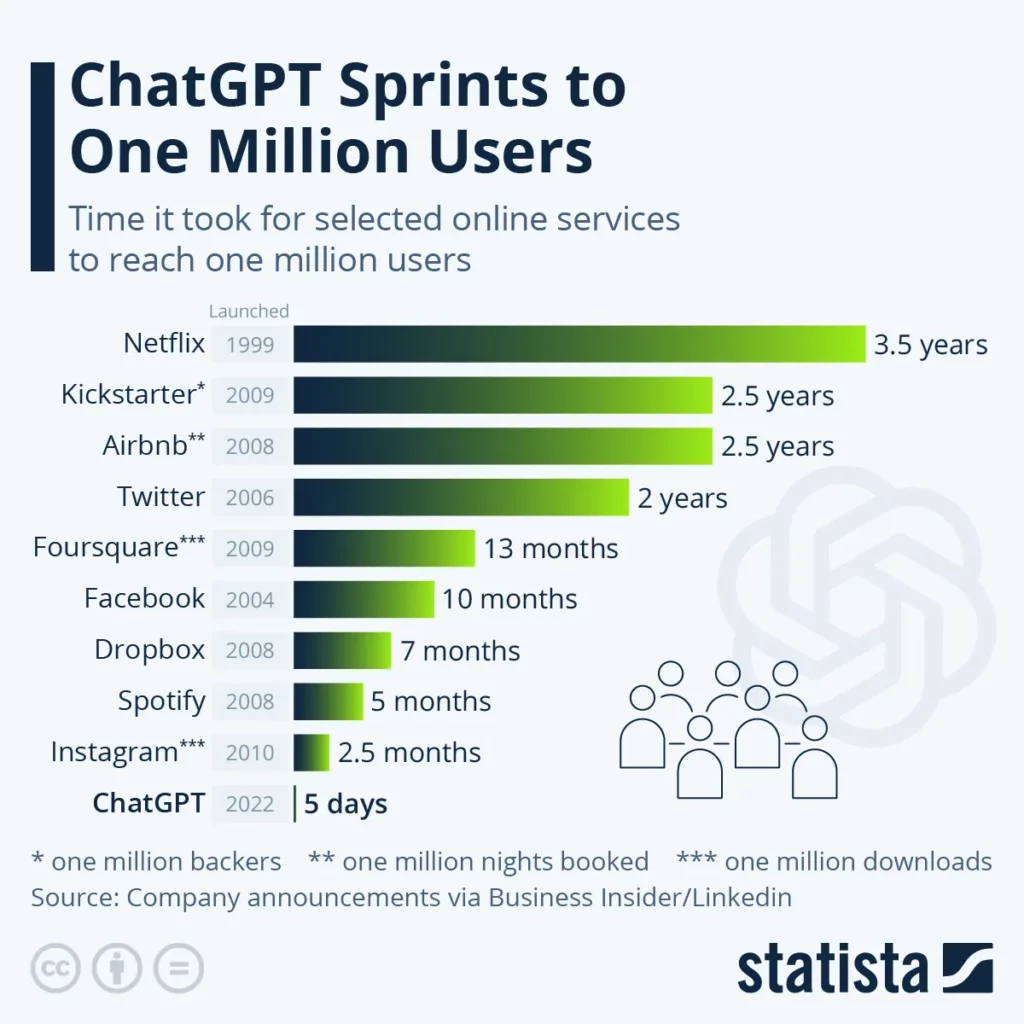
Source: Statista
ChatGPT broke the Internet in 2022, reaching 1M users in just five days, with growth far eclipsing generationally-defining consumer platforms like Instagram.
It’s worth noting that this heavily-trafficked chart is a little misleading, for a variety of reasons, including that it directly compares different types of user engagement, e.g. app downloads vs. unique usage. It also compares 2022 Internet usage and interaction paradigms to those of 2010. Quibbles aside: it’s pretty clear that we just witnessed AI’s breakout consumer moment.
I wrote in December about the rise of the conversational interface. We’re seeing that user experience paradigm take hold in a number of new platforms, including social experiences, search, and more. But beyond LLM-based chatbots, we should expect many net new consumer experiences leveraging generative models to be born in this new era.
#6: Industry is outpacing academia in driving AI breakthroughs.
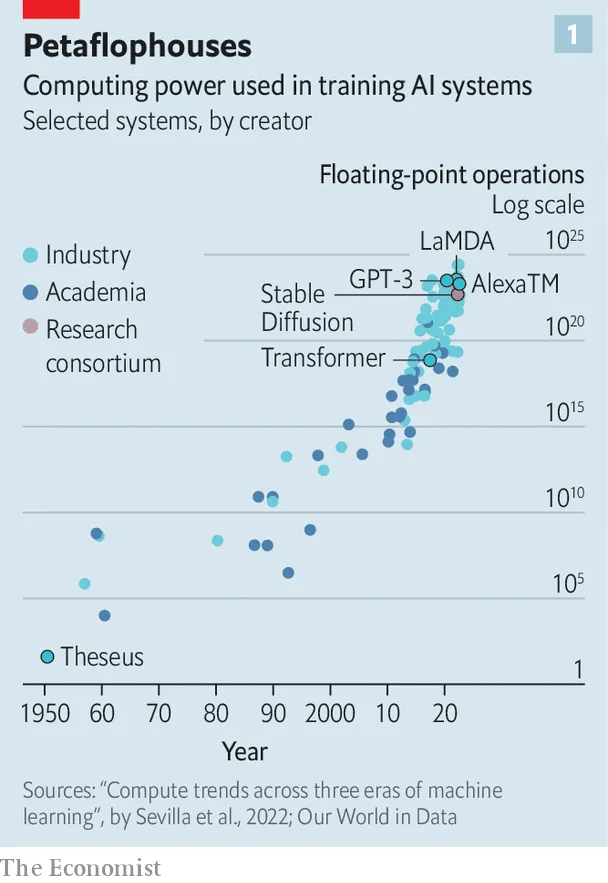
Source: The Economist, “The race of the AI labs heats up”
It’s not a new trend per se, but in 2023, industry’s research muscle continues. The Economist chart above outlines compute trends over time, noting both the massive compute requirements of state-of-the-art AI systems over the past five years, and industry’s centrality in producing these systems. That’s no coincidence, as the compute, data, and talent to build these models doesn’t come cheap.
The Verge calls this AI’s “era of corporate control.” Indeed, unlike previous major breakthroughs like the Internet or even nuclear technology, AI has effectively been “born commercial.” That matters a lot. Despite companies’ claims of commitment to basic research, industry R&D has fundamentally different motivations than publicly-funded R&D. As a society, are we missing out on important basic research because of industry’s leading role?
#7: There is growing regulatory scrutiny of artificial intelligence applications.
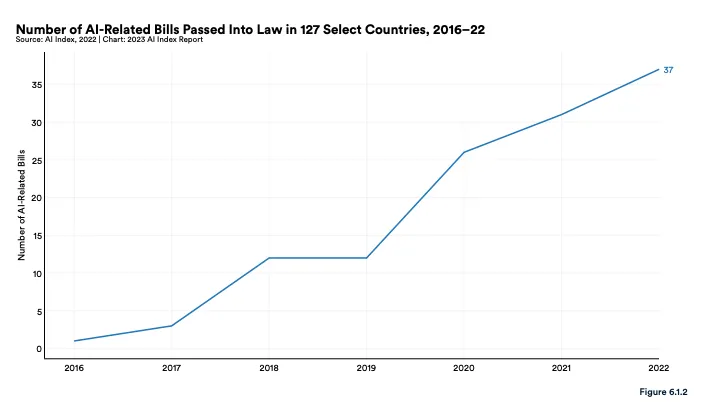
Source: Stanford HAI, 2023 AI Index Report
The age of unbridled experimentation in AI may be over.
Since 2016, a total of 123 AI-related bills have been passed by legislative bodies globally (from a select 127 countries). Expect this number to increase. Earlier this month, the U.S. Department of Commerce invited public comment on AI regulation. The EU is also considering omnibus AI legislation called the Artificial Intelligence (AI) Act. Last week, Alphabet CEO Sundar Pichai underscored the need for global AI regulatory frameworks. The Future of Life Institute’s recent open letter calling for slowdown in powerful AI system development has likely added urgency and momentum to these debates.
In this context, AI governance will once again be a hot-button issue, raising questions of tradeoffs between speeding up and slowing down.
#8: FLOP era: GPUs are in seriously short supply.
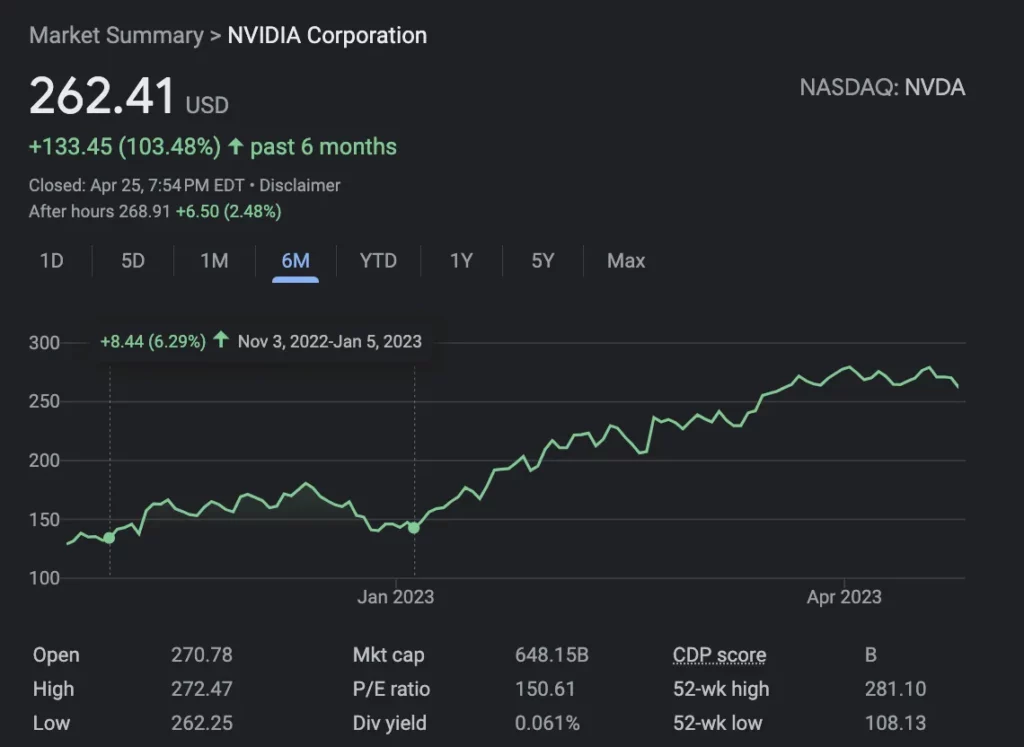
Source: Google Finance, 4/25/2023
#9: Agents, everywhere.
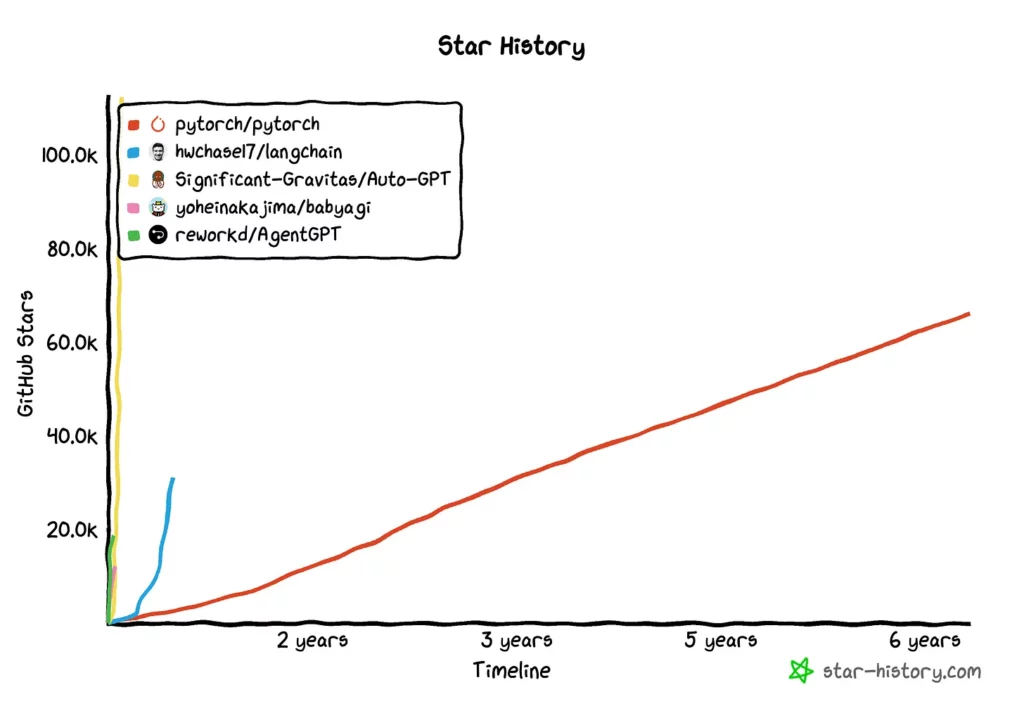
Source: star-history.com
Over the past few weeks, task-driven autonomous agents like AutoGPT, AgentGPT, and BabyAGI have become enormously popular. AutoGPT, for instance, recently surpassed Pytorch in GitHub stars. Growth of popular agents has surpassed libraries like Langchain, which itself previously broke growth records!
Momentum aside, it is not clear how useful these experiments are in production. Most OSS agents seem like snazzy demos right now, rather than real products or businesses. There are also questions of differentiation and durability as LLM providers consider building up the stack. The notion of powerful, sometimes unpredictable models executing digital tasks without guardrails also raises all kinds of AI safety questions.
#10: Great power competition continues to extend into the world of AI.
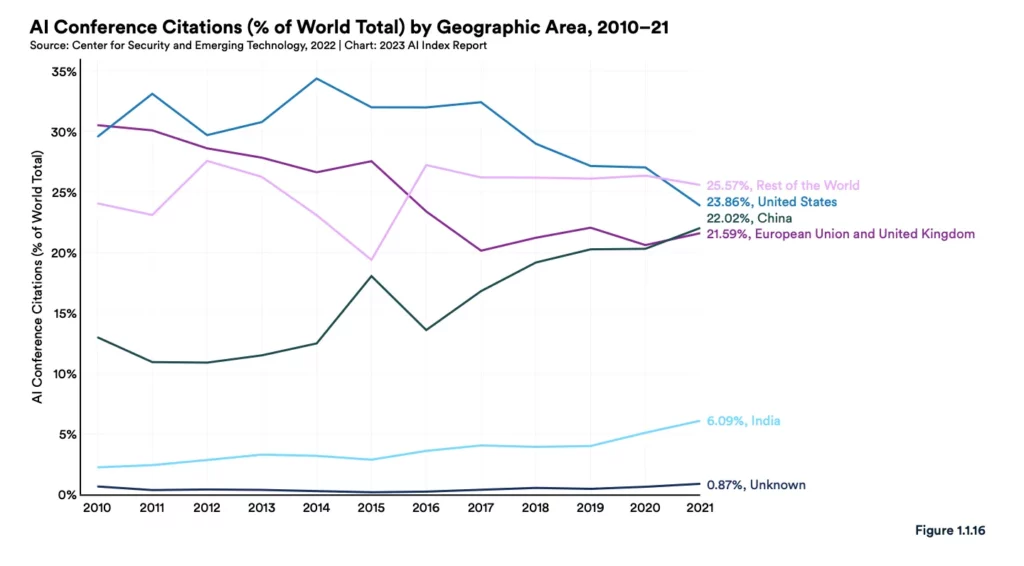
Source: Stanford HAI AI Index Report 2023
AI research has long been an arena for great power competition (remember that 2017 Putin quote?). The U.S. and China in particular continue to compete in AI R&D and commercialization. The prevailing theory has historically been that the U.S. leads in true innovation, even if China may produce a higher volume of research. This chart from Stanford’s latest AI Index Report suggests that that gap may be narrowing — though the U.S. leads China in AI conference citations, the gap in 2021 was slim and getting slimmer. Expect AI research to be an area of continued focus from both national governments, and competition (and collaboration) ongoing areas of friction.
Radical Reads is edited by Leah Morris (Senior Director, Velocity Program, Radical Ventures).