AI startups are getting a lot of attention. While the broader venture investing market has significantly contracted over the last twelve months and venture capital (VC) fundraising just hit a nine-year low, it seems that everyone and anyone is trying to invest in “generative AI” companies these days.
Stability AI raised a $100 million round at a $1 billion post-money valuation in October. Anthropic is in the late stages of raising $300 million at a $5 billion post-money valuation right now. General Catalyst is allegedly leading an investment round into Adept, and a16z is allegedly leading a $250 million investment round into Character.AI, both at $1 billion post-money valuations. What is most shocking about all of the above fundraising rounds, however, is that all of these businesses are pre-revenue.
Never in venture history have pre-revenue businesses raised this much money at such jaw-dropping valuation multiples, and this is happening in the face of the biggest technology valuation pullback of the last twenty years. Why? Is this simply the result of a record number of venture capitalists trying to deploy a record amount of dry powder in short order? Is “generative AI” the biggest bubble since the dot-com crash? Or, is there actually something fundamentally different about AI businesses driving a de-coupling from traditional valuation metrics and the broader macroeconomic conditions?
While there is an outsized amount of buzz and frothiness in the AI universe today, a fair amount of this is likely being driven by the underlying economics of AI businesses. What is the primary culprit? A hint:
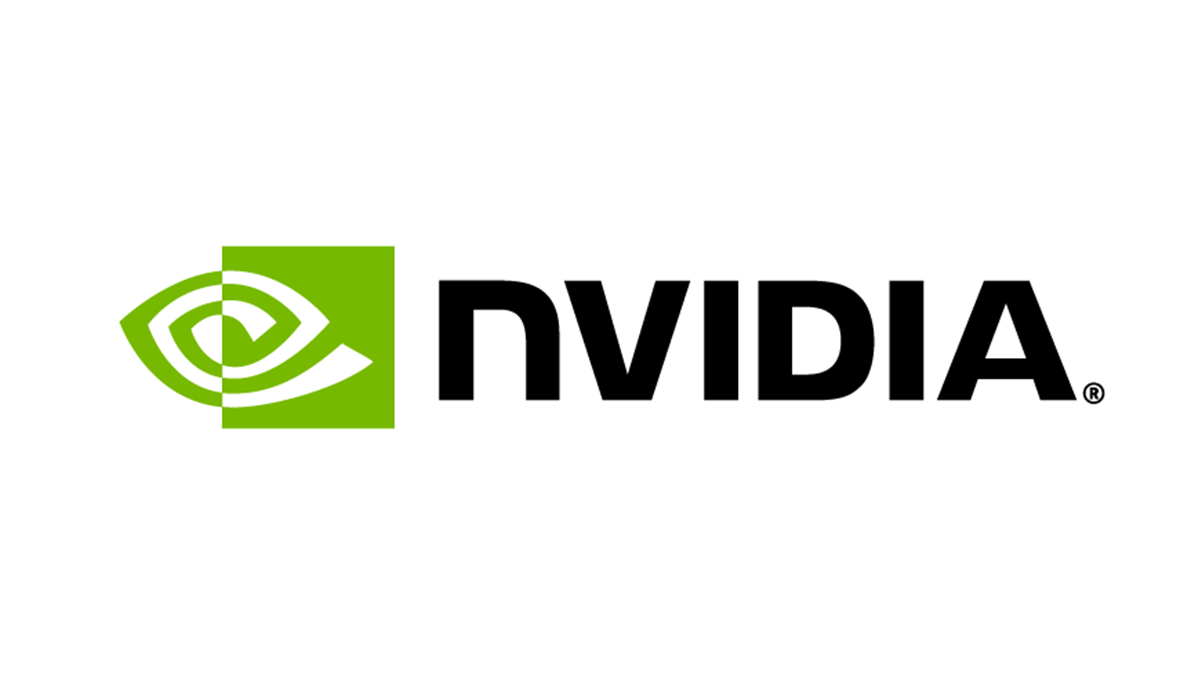
Returning to Valuation Math
Early stage venture valuations tend to be driven by the amount of funding that a company needs in order to reach their next milestones. Traditionally, this math was fairly simple:
- Burn rate = salaries + overhead (rent, software licenses, employee laptops, etc.)
- Runway (typically) would be two years
- At the Seed stage, the “milestone” required to ultimately raise a Series A was $1 million of ARR (while showing sufficient growth)
In practice, this used to mean that a Seed round would typically shake out to be an approximately $2 million investment $6-8 million pre-money valuation. Recently, these numbers have probably crept up closer to $3-5 million rounds ($10-20 million pre-money) as startup salaries have increased. However, for AI businesses, there is often a third variable at play in the burn rate: compute / model training spend.
GPT-3 originally cost $12 million to train from scratch (ignoring all prior R&D spend). While the cost of training a model of this quality has come down significantly, annual spend has not as AI companies continue to push what is the cutting edge forward (we have heard that the training spend for GPT-4 has been approximately $40 million). So far, as has been well documented, the big early winners in the AI space race have been companies like Nvidia who power model training. These businesses will likely continue to feast on VC funding rounds, but eventually, most of the value creation will shift to AI companies themselves.
While AI startups do not need to compete head-to-head with OpenAI, they do need to figure out a way to provide comparable levels of quality. Often, this means training their own models.
The Cost of Funding Foundation Model Companies
If a business is looking to build its own model (in whichever domain they are targeting), they are often referred to as building ‘Foundation Models’, which build Transformer-based models in-house. Radical Ventures has invested in quite a few of these businesses including Cohere, Covariant, and Twelve Labs, as well as others in stealth. We believe that these companies are building globally significant businesses in each of their domains.
A common characteristic of Foundation Model companies is their need for significant compute to train models. In general, at the Seed/Series A stage, we have seen businesses in this category spend anywhere from $1 million to $50 million or more per year on model training once they are fully operational (not including serving costs). As one can imagine, this throws a fairly large wrench into the typical venture capital valuation math.
Assuming we take the midpoint of the above figure, and that it takes a company approximately six months to be fully ramped, the “burn rate stack” would look something like this for two years of runway:
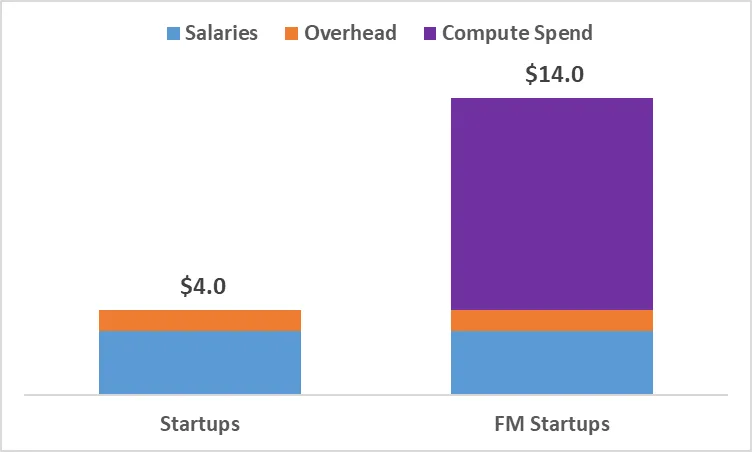
As one can imagine, this affects how an early-stage venture is valued. Leveraging the framework laid out last week (25% round dilution), if these example businesses got funded, the traditional startup would raise at $12 million pre-money/$16 million post-money, and the Foundation Model startup would raise at $42 million pre-money/$56 million post-money: a bizarre outcome for what is clearly a less capital efficient business.
Deeper Down the Rabbit Hole
Beyond increased burn rates, however, Foundation Model companies also tend to have different milestones than traditional startups. Unlike a traditional startup, which can simply write code, ship a product, and iterate on customer feedback, Foundation Model companies need to spend more time building and training their models in order to get a product to a position in which it is viable and ready to be used. Often, this can take multiple years, millions of dollars (or, in the examples in the first paragraph hundreds of millions of dollars), and several iterations before products are good enough for companies to charge customers to use them. In short, when we think about milestones, it is not fair to apply the traditional startups metrics listed above to these companies. Almost none of these companies will have $1 million of annual recurring revenue (ARR) at the time of their Series A, and many will spend tens (or hundreds) of millions before charging their first customer anything.
In practice, we have seen these types of companies target “product milestones,” instead of revenue milestones, as they look to raise Seed and Series A rounds. These milestones help to keep companies accountable as they progress and grow. A Foundation Model company that cannot hit any of it’s own relevant milestones (e.g., world-class performance in their domain) will not be able to raise a subsequent funding round, the same way that a traditional SaaS company will not be able to raise if they miss their revenue targets.
Eventually, will the Math Matter?
In a previous article, the opposite point was made: “eventually, the math is going to matter.” Let’s revisit the good outcome from the previous article (Scenario A):
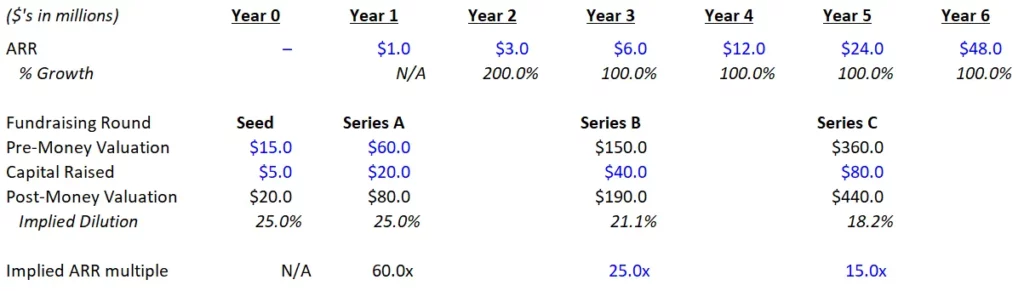
In this example, this company raised a large Seed and Series A, and therefore needed to grow extremely quickly to raise a successful Series B (in this case, to get to $6 million of ARR). If we apply the same principles, but to a Foundation Model business (which likely will not have any revenue until approximately year 3), the math quickly breaks down:
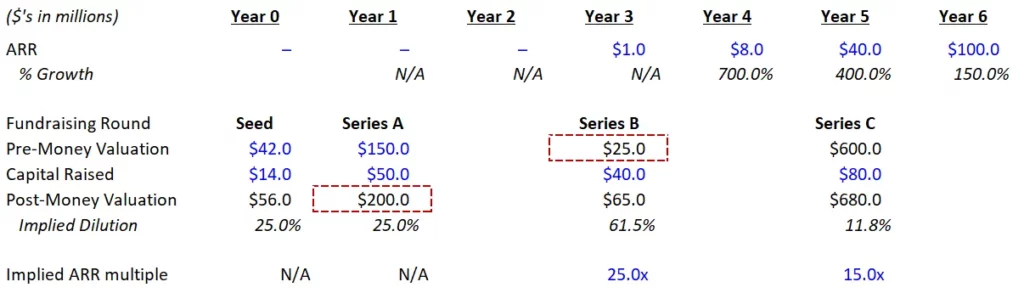
Yikes – a down round going from $200 million to $25 million would clearly kill a business. Are these really just awful businesses? Or is this a similar situation to hardware businesses, which have long been debated as not venture-backable due to their capital intensity?
Arguably, Foundation Model businesses are in a different boat altogether: while they are capital intensive up front, they can scale at rates fast enough to justify the capital investment. As some folks might have noticed in the above example, the Foundation Model business actually passes the Software business in ARR in year 5, and therefore raises at a higher Series C valuation. If this hypothetical company did manage to survive, it would become the larger (and more valuable) business in the long run.
The Magic of Exponential Growth
Some may argue that the example given above is unrealistic because a company cannot 8x its revenue year over year (YoY). Anyone could justify their model by using higher growth figures to make the math work. This is true. But, this example is enough of a prompt to get the thought experiment rolling. If you are going to invest in Foundation Model companies, you have to believe that they will grow faster than businesses ever have before.
Professor Albert Bartlett famously said, “The greatest shortcoming of the human race is our inability to understand the exponential function.” Professor Albert Einstein famously said, “Compound interest is the eighth wonder of the world. He who understands it earns it…. he who doesn’t… pays it.”
The simple point is exponential growth is a big deal and is often an unintuitive concept to wrap our brains around. It is exceptionally rare to actually see it in action, but we believe we are already seeing it in the rate of AI adoption:
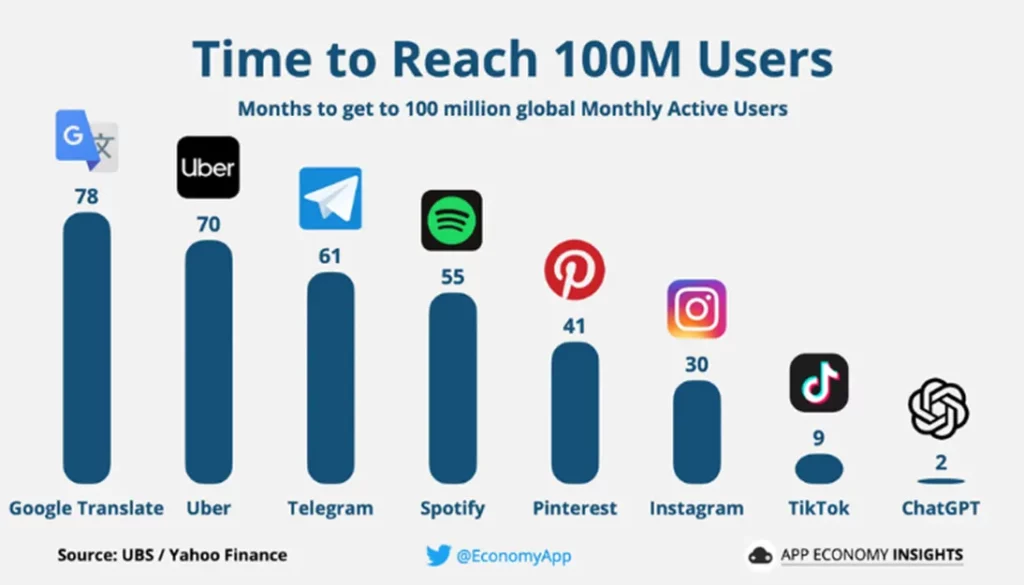
Above is a graphic that outlines the number of months it took for ChatGPT to reach 100 million users globally. Despite the viral adoption of ChatGPT, we are in the very early innings of AI adoption. We expect to see many products reach massive adoption numbers given the recent advances in Foundation Models and the wide application of large language models (LLMs) to most software and so many business functions.
As we see unprecedented growth in these types of technologies, we should also expect to see extraordinary growth in some of the core Foundation Model companies that emerge. For these startups, gone are the years of “triple, triple, double, double” annual growth: we will see a new, higher standard emerge for the very best businesses.
Putting it all together, we expect some foundation model companies to present a “deeper J-curve” or “hockey stick” than what we have seen in past startups, as more capital is required up front to unlock exponential growth on the back end.
The Time is Different
There are two major points here that you have to accept as true, however, to believe this hypothesis:
- AI solutions are “10x better” than legacy software solutions and are going to grow much faster than traditional SaaS startup benchmarks (i.e., faster than 2-3x YoY). In this scenario, you would have to believe that the shift towards AI will be the disruptive “platform shift” that we see in the technology space once every 15 to 20 years (for example the shift to Internet, shift to mobile, etc.).
- The sheer cost and scarcity of compute resources will create a strategic moat for startups who raise rounds quickly and train their models first. While the cost of compute is decreasing, annual compute spend is not. Historically, the “capital as a moat” strategy has never worked well, but traditionally that is money being spent on sales and marketing promotions. If compute spend is more defensible, it may become a strategic advantage (similar to how building a factory can be a durable strategic advantage for manufacturers).
If you do not believe either of those will be true, it is safe to say that you would disagree with the above hypothesis. Stating that “this time is different” is widely pointed to as one of the most common investing pitfalls in history. Therefore, if you are going to make a bet on that, you need to really believe it.
So What Does it All Mean?
TL;DR: AI businesses that build their own models (Foundation Models), are more capital-intensive upfront, but offer the promise of exponential growth in the long run. So far OpenAI’s ChatGPT is the only real example of that coming to fruition.
Thinking about what this all means, a few things come to mind:
- If these businesses do not end up experiencing outsized growth, they will be subject to a challenging and difficult period and may look toward an exit strategy.
- The companies that do execute on the promise of exponential growth will reach a size and scale that almost no startups have before. For the first time in the last decade or two, it feels like the current Big Tech companies are actually vulnerable to disruption (see: Alphabet losing $100B in market cap in a single day after investors worried they were falling behind in the AI race).
- The “deeper J curve” hypothesis requires later-stage funding rounds in this category to be executed using the same principles of early-stage venture rounds, with valuations disjointed from business fundamentals. While this sounds like a tall order, so far, investors seem to be willing to play that game (it is worth noting, though, that many of the funds leading these rounds are the growth investing arms of traditional VC funds, as opposed to pureplay growth investors).
- While it has historically been disregarded as unsustainable, there is likely some validity to the “capital as a moat” strategy that has always been widely debated. As potential employees and customers acknowledge the outsized risk in the category, we are increasingly seeing a draw toward companies that have already raised massive venture funding rounds and therefore have significant runway. These companies could be rebranded as having a “Field of Dreams” fundraising strategy (i.e., “If you build it, they will come.”)
- Inevitably, we are bound to see significant amounts of disruption in the coming years with increasing amounts of AI adoption. While in general AI will likely bring more good than harm into the world, there will be legacy businesses that do not survive the transition. Most probably, businesses with strong distribution advantages (e.g., proprietary sales channels) are the least likely to be disrupted.
A Brief Note on Other Types of AI Businesses
It should be noted that most AI businesses are not Foundation Model businesses themselves, and such do not require the same level of capital intensity. Some businesses are purely applied AI products and services that leverage other Foundation Models technology and use it to power their tailored offerings in specific end markets (e.g., marketing copy generation). These businesses can sometimes experience the best of both worlds and benefit from minimal capital intensity and exponential adoption (e.g., Jasper AI), but sometimes struggle with building defensibility around their business and preventing churn.
Others take a midpoint approach and focus on significantly “fine-tuning” a Foundation Model’s technology to their specific customer or use case, often with a unique and proprietary dataset: mitigating some of the capital intensity while still maintaining the defensibility of their offering.
Another category sees businesses building tooling around Foundation Models (LLMOps/FMOps, etc.). These companies do not need to train their own models while still being able to ride the exponential growth in the broader category (often called “picks and shovels” plays). These are experiencing viral developer adoption right now, but there is also an outstanding question about how durable these offerings are in the long run, and if Foundation Model businesses will just replace these tools with their own.
What we are seeing, however, is all three of these business types trying to raise at Foundation Model valuations given the hype in the space. This is not logical, given the compute spend, the driving factor for increased capital needs is much lower. As tourist investors enter the space, we suspect that several of these businesses will get funded and may fall into a valuation trap. As exciting as the space is, if investors are not careful, companies of today’s vintage may go to zero in the coming years.
AI News This Week
-
The Power List: top 10 AI trailblazers (Macleans)
Macleans lists the top innovators and iconoclasts placing Canada at the forefront of artificial intelligence. In this year’s list, Radical Ventures and Radical portfolio company founders claimed half of the spots. The list includes Radical Ventures co-founder and Managing Partner Jordan Jacobs, his Vector Institute co-founder and Radical investor Geoffrey Hinton, and the founders of Radical portfolio companies Waabi (Raquel Urtasun), Cohere (Aidan Gomez, Nick Frosst and Ivan Zhang), and Xanadu (Christian Weedbrook). Tomi Poutanen, Radical co-founder and co-founder of Signal 1, also made the top ten list for innovations in healthcare.
-
GPT-4 is here: what scientists think (Nature)
Four months after the release of ChatGPT, OpenAI released the much-awaited GPT-4. The updated model is multimodal (not just image-to-text) and accepts image inputs including pages of text, photos, diagrams, and screenshots. However, despite pre-launch rumours, there is no text-to-video capability. A new type of input called a system message was added to instruct the model on the style, tone, and verbosity to use in subsequent interactions. The model’s performance against a variety of AI benchmarks was published by OpenAI, but minimal information is available on the data used to train the system, its energy costs, and the specific hardware or methods used to create it.
-
Listen: AI search at You.com with Bryan McCann, co-founder & CTO of You.com (Changelog)
Neural search and chat-based search are all the rage right now. Radical Ventures portfolio company You.com was the first to incorporate chat with search.. In this podcast episode, You.com co-founder and CTO Bryan McCann shares insights on the mental model of Large Language Model (LLM) interactions and practical tips related to integrating LLMs into production systems.
-
What can AI art teach us about the real thing? (The New Yorker)
The legitimacy of AI generated images remains controversial in the art world, despite the process being underpinned by art history. Images are generated from a catalogue of existing works as a result of a dialogue between the prompter and the prompted. Users then critique the output and iteratively adjust the prompt. A human’s ability to detect errors and subtle emotional cues in an image is astounding. Online discussions this week surrounding one of the latest image generation models illustrate our ongoing dialogue with AI systems in our pursuit to capture “realness.”
-
These researchers used AI to design a completely new ‘animal robot’ (Scientific American)
“Xenobots” are living, swimming self-powered robots that measure less than a millimetre across. They are designed by AI and built out of frog (Xenopus Laevis) stem cells. Computer scientists and developmental biologists worked together to develop the technology which could open new medical frontiers. The researchers envision making a fully biological robot that could go inside the body and perform functional tasks in place of high-risk surgeries. In parallel, the team is studying how cells assemble to better understand neurological intelligence and treat diseases.
Radical Reads is edited by Leah Morris (Senior Director, Velocity Program, Radical Ventures).